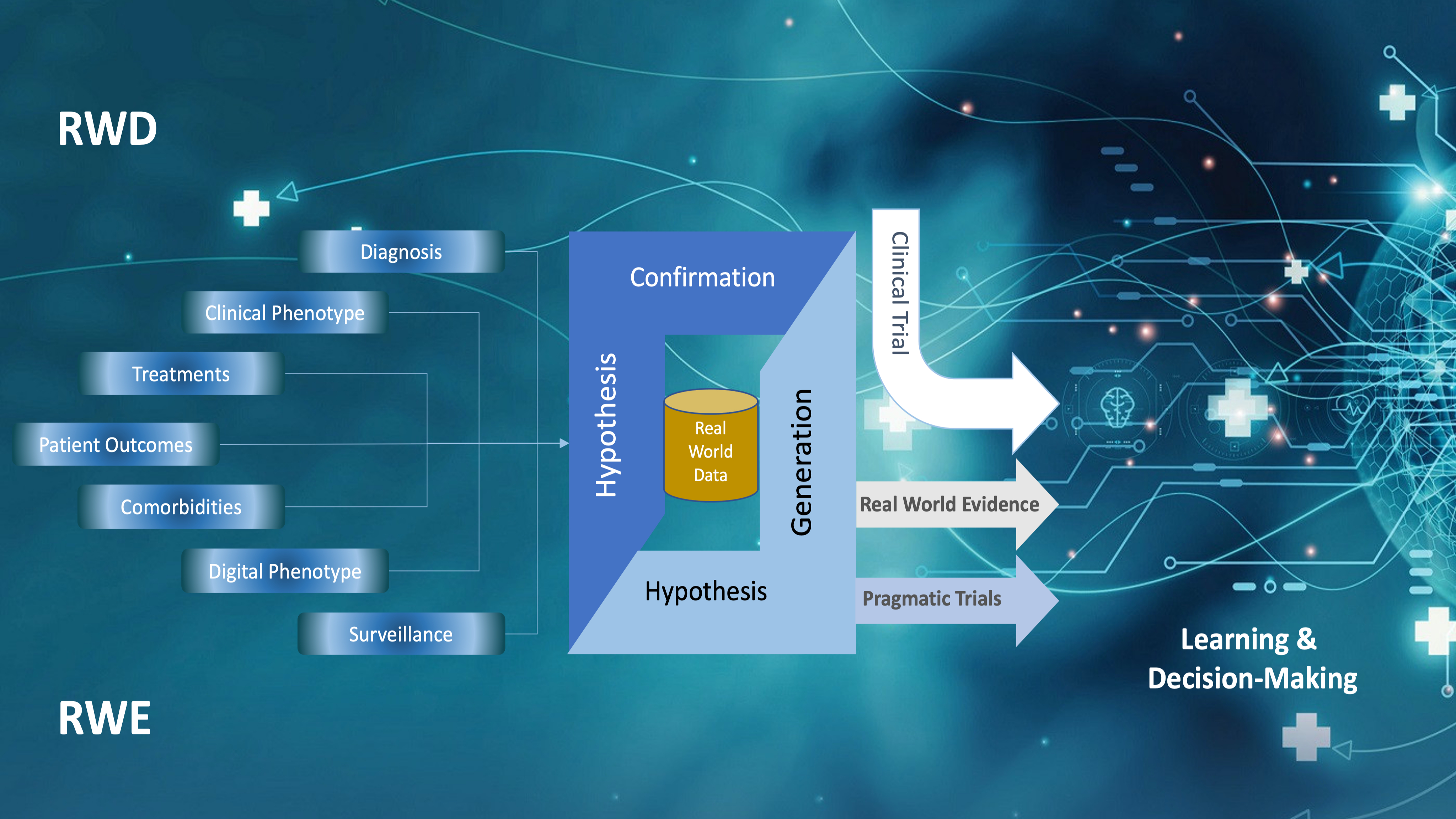
Transforming Healthcare Decision-Making with RWD & RWE
Introduction
Real World Data and Real World Evidence are becoming crucial in healthcare research, policy-making, and clinical decision-making. These concepts are increasingly used to complement traditional clinical trial data, providing a more comprehensive understanding of treatment outcomes in broader patient populations, including those who are often excluded from clinical trials. This article explores the definitions, sources, uses, and challenges associated with RWD and RWE.
Real World Data and Real World Evidence are transforming the landscape of healthcare. By providing insights based on actual patient experiences, these tools offer a more holistic, personalized approach to medicine. As technologies and methodologies continue to evolve, RWD and RWE will play an even greater role in improving patient outcomes, informing clinical practice, and shaping policy decisions. However, addressing challenges related to data quality, privacy, and biases will be crucial to maximizing the potential of these valuable resources.
Defining Real World Data (RWD)
Real World Data refers to data collected from a variety of sources outside the controlled environment of traditional clinical trials. Unlike data derived from randomized controlled trials (RCTs), which are highly structured and involve strict eligibility criteria, RWD reflects the diversity of patients encountered in everyday clinical practice.
Sources of RWD include:
Electronic Health Records (EHRs): Patient health data from hospitals, clinics, and outpatient settings, including diagnoses, medications, treatment plans, and outcomes.
Claims and Billing Data: Information from insurance claims, which includes data on diagnoses, procedures, and treatments used for reimbursement purposes.
Patient-Reported Outcomes (PROs): Data collected directly from patients regarding their health status, quality of life, and treatment satisfaction.
Registries: Databases that track outcomes in specific patient populations, such as those with rare diseases or undergoing particular treatments.
Mobile Health Devices: Data from wearable devices and apps that monitor health metrics such as physical activity, heart rate, or glucose levels.
Social Media and Patient Forums: Informal sources of data where patients share experiences and discuss treatments.
Defining Real World Evidence (RWE)
Real World Evidence refers to the insights and conclusions drawn from the analysis of RWD. RWE can be used to evaluate the effectiveness, safety, and value of treatments or interventions in a real-world setting. While clinical trials often focus on efficacy in a controlled population, RWE focuses on effectiveness in a broad, diverse patient population.
RWE is produced through various study designs, including:
Observational Studies: These studies analyze RWD without manipulating variables, allowing researchers to observe outcomes based on real-world exposure to treatments.
Retrospective Studies: Researchers use existing data to examine past outcomes, looking at trends and associations between treatments and health outcomes.
Prospective Cohort Studies: These studies follow patients over time to assess long-term treatment effects or health outcomes.
Natural Experiments: These take advantage of situations where different groups receive different treatments due to external factors (e.g., policy changes or regional differences in treatment practices).
Uses of Real World Data and Real World Evidence are increasingly used in various aspects of healthcare:
Regulatory Decision-Making: Regulatory agencies like the FDA and EMA use RWE to supplement clinical trial data when approving new drugs or treatments. RWE can support label expansions, post-market surveillance, and risk-benefit analyses.
Clinical Practice: RWD helps clinicians make informed decisions based on the experiences of similar patients rather than relying solely on clinical trials, which may not fully represent the diversity of the population.
Health Technology Assessment (HTA): RWE can inform cost-effectiveness models, helping policymakers determine the value of new treatments compared to existing options.
Drug Development: Pharmaceutical companies use RWD to identify unmet medical needs, discover biomarkers, and refine clinical trial designs by understanding the patient population’s characteristics and treatment outcomes.
Post-Market Surveillance: RWD is vital for monitoring long-term safety and effectiveness after a drug or device enters the market. It can detect rare side effects and signal new uses for existing treatments.
Patient-Centered Outcomes: Patient-reported outcomes collected from RWD can provide a more holistic view of how treatments affect quality of life, adherence, and other non-clinical factors important to patients.
Benefits of Real World Data and Real World Evidence
Diversity of Patient Populations: Unlike clinical trials, RWD captures data from a more diverse patient population, including different age groups, comorbidities, socioeconomic statuses, and ethnic backgrounds, making it more generalizable to the broader population.
Faster Decision-Making: RWD enables quicker decision-making as it provides real-time data and insights. This is particularly valuable in responding to urgent public health issues or in assessing new treatments post-launch.
Cost-Effective: Using RWD is often less costly than conducting large-scale clinical trials. It leverages existing data, thus reducing the time and financial investment required for primary data collection.
Identifying Long-Term Effects: Clinical trials typically focus on short-term outcomes, but RWD can help capture long-term effects of treatments, including rare adverse events or delayed therapeutic responses.
Personalized Medicine: By analyzing RWD, healthcare providers can better understand which treatments work best for specific patient subgroups, facilitating personalized or precision medicine.
Challenges and Limitations
Despite its numerous advantages, RWD and RWE also come with challenges:
Data Quality and Completeness: RWD comes from various unstructured and heterogeneous sources, making it prone to issues like missing data, inconsistencies, and inaccuracies. Ensuring data quality is critical for producing reliable RWE.
Bias and Confounding Factors: Unlike randomized trials, observational data can be subject to biases such as selection bias, reporting bias, and confounding factors that can distort the findings. Careful statistical methods are needed to account for these challenges.
Privacy Concerns: Given the sensitive nature of health data, maintaining patient privacy and complying with regulations like the GDPR and HIPAA is essential when collecting and analyzing RWD.
Regulatory Acceptance: While regulatory agencies are increasingly using RWE in decision-making, there is still some skepticism about the robustness and generalizability of findings based on RWD, especially when compared to RCTs.
Data Integration: Integrating data from multiple sources (e.g., EHRs, claims, wearables) can be technically complex and time-consuming. Standardization of data formats is essential for meaningful analysis.
The Future
The future of Real World Data and Real World Evidence is bright, with several developments likely to enhance their role in healthcare:
Advanced Analytics: With the advent of machine learning and artificial intelligence, the ability to process vast amounts of RWD quickly and accurately is improving. These technologies can help identify patterns and predict outcomes more effectively.
Data Linkage: Linking data from different sources, such as EHRs, claims, and registries, can create a more comprehensive view of patient journeys, providing deeper insights into treatment efficacy and safety.
Patient-Centered Research: Greater involvement of patients in data collection, through wearable devices and digital health platforms, will ensure that RWD better reflects patient experiences and preferences.
Global Collaborations: As health systems worldwide become more interconnected, global data-sharing initiatives will enhance the quality and quantity of RWD, facilitating broader analyses and faster innovation.
Regulatory Frameworks: As RWE becomes more integral to healthcare decision-making, regulatory agencies are likely to establish clearer guidelines on how to use RWD for drug approval, reimbursement, and safety monitoring.